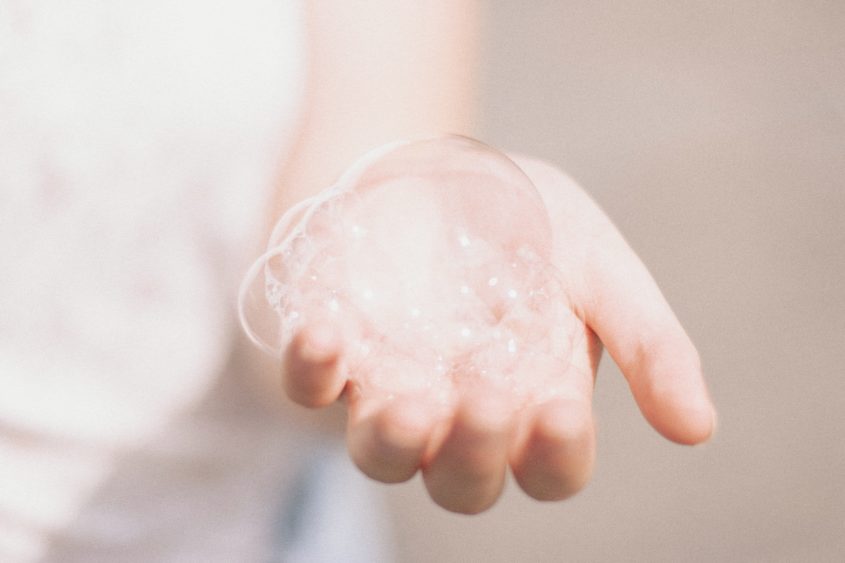
Bias Laundering in Interviews
Bias Laundering is a phrase most commonly used to describe machine learning models. Because Machine Learning is trained on data from existing systems, it commonly replicates and institutionalizes any bias already existing in that system. The “laundering” happens when that algorithm is then trusted to be objective or neutral, thereby shielding the bias built into the system as inevitable and correct.
During an interview, evaluating candidates for vague qualities of questionable utility can have the same impact. One examples is consideration of how “excited” the candidate seems to be in the interview. Unless your interview rubric evaluates for this trait in every candidate and judges them all by the same, unambiguous standard, you’re probably working backwards from a feeling rather than truly evaluating the candidate.
For example, in software, it’s common for women to be rated lower in interviews for not being bubbly enough. This is something that men are essentially never expected to perform, but when it comes time to collect interview feedback, most interviewers aren’t going to say something like “Women should smile more!” Instead, they’ll say something like “she didn’t seem very excited about the opportunity”.
What’s usually happening here is the interviewer rationalizing their gut instinct. They feel that the candidate wouldn’t be a good hire but the rubric is telling them they would, so they work backwards from the answer through mechanisms that are ambiguous enough to catch anybody or nobody.
The impact is your team missing out on great candidates, reinforcing undesirable preferences, and creating a less inclusive environment on your team1.
How to Minimize Bias Laundering
The first step to reducing the amount of bias laundering that happens in your interviews is to start identifying it in yourself. Look for and recognize the slightly unmoored feeling of having your official rubric pointing in one way but feeling like the answer should be different. Try to understand where it’s coming from. Think about common forms of bias that the candidate might experience, re-evaluate them against the agreed-upon rubric, and if you can’t find an unambiguous reason to go with your gut, stick to the answer your rubric gives you.
Identifying it in other folks is harder, but keeping an eye out for phrases like “I can’t put my finger on why” and try to push interviewers away from any evaluation that doesn’t unambiguously implicate the agreed upon rubric.
For instance, if being collaborative is a key value at the company and my colleague is saying they don’t think the candidate would be collaborative enough because they weren’t friendly enough in the interview, I’d first want to identify if the candidate was being rude, hostile, or otherwise difficult. Was there an actively negative vibe — something we’d want to avoid regardless of who was showing it off? If so, rephrase the criticism in more direct terms. If not, I’d want to learn how the lack of collaboration would manifest.
If the interviewer said, for example, they’d be less likely to go ask this person for help because they weren’t friendly enough, it might be worth exploring whether that’s actually the candidate’s issue or indicative of the interviewer having a less inclusive mindset than would be optimal2.
A less confrontational approach would be to re-run the evaluation criteria. Confirm that the candidate was responsive to questions, took feedback well, etc. If so, suggest that it might just take some time to get used to their particular style and remind the interviewer that adding someone to the team likely requires a bit of compromise from everyone to ensure the team continues to perform well.